Building Better Data Platforms with CI/CD
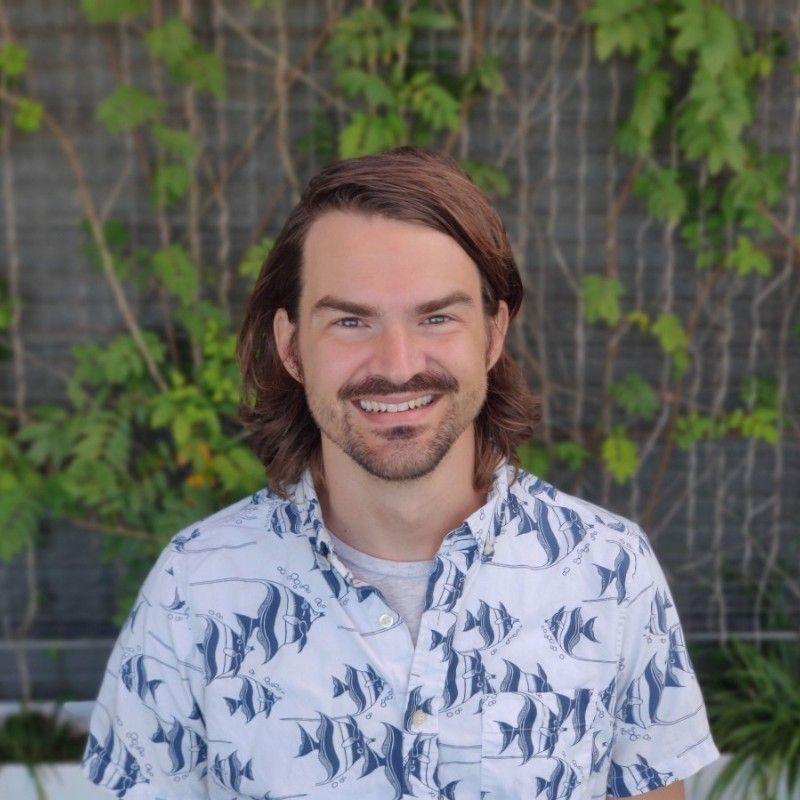
In modern data work, the gap between development and production environments creates significant challenges. Data pipelines that run smoothly in development often fail in production because of differences in data volumes, schema variations, or system dependencies. Continuous Integration and Continuous Delivery (CI/CD) practices offer a structured approach to bridging this gap, enabling teams to build more reliable, adaptable data systems. By implementing automated testing, deployment, and monitoring processes, data engineers can detect issues earlier, deploy with greater confidence, and respond more quickly to changing requirements which ultimately unlocks more value from their data.
The Fundamentals of CI/CD
Continuous Integration and Continuous Delivery (CI/CD) evolved as a solution to a common problem in software development. Before CI/CD, developers would work independently for weeks or even months before merging their code. This process, known as integration, was often painful, time-consuming, and revealed numerous conflicts between different developers' work.
Continuous Integration emerged as a practice where developers merge their changes back to the main branch frequently, often multiple times per day. Each merge triggers automated builds and tests to detect problems early. This approach significantly reduces integration problems and allows teams to develop cohesive software more rapidly.
Continuous Delivery extends this concept by ensuring that code is always in a deployable state. After passing automated tests, the code is ready to be deployed to production at any time, though the actual deployment might still require manual approval.
Some teams take this a step further with Continuous Deployment, where every change that passes all tests is automatically deployed to production without human intervention.
How CI/CD Works in Practice
The journey of code from a developer's computer to production follows a structured path in a CI/CD environment. When a developer completes a feature or fix, they push their code to a shared repository. This action triggers one or more CI/CD pipelines, which typically includes several stages.
First, the code is built, converting human-readable source code into executable software and ensuring all dependencies are properly resolved. Next, automated tests run to verify the code works as expected. These tests might check individual functions (unit tests), interactions between components (integration tests), or entire user workflows (end-to-end tests).
If the tests pass, the built application can move to staging environments that closely mimic production. Here, additional tests might run to catch issues that only appear in live settings. Finally, with sufficient confidence in the build’s quality, it's deployed to production where it becomes available to users.
Throughout this process, the CI/CD system provides feedback to developers. If any stage fails, the team is immediately notified, allowing them to address issues quickly before they affect users. Version control strategies like GitFlow help teams manage this workflow by organizing code into feature, development, and production branches.
CI/CD for Data Engineering
CI/CD for data applications comes with its own set of challenges. Unlike traditional applications that primarily process business logic, data pipelines rely on and manipulate large volumes of stateful data from various sources. These pipelines must handle changing data schemas, varying data quality, and complex transformations while maintaining reliability.
The fundamental principles of CI/CD still apply to data engineering, but with adaptations. Data pipeline tests must verify not only that the code runs correctly but also that it produces the expected data transformations. This requires representative test data that reflects the patterns, edge cases, and volumes seen in production.
Environment management becomes particularly important for data pipelines. Development, testing, and production environments need isolated data stores to prevent test operations from affecting production data. Configurations must adapt to different environments while maintaining consistent pipeline behavior.
Data validation emerges as a critical component of CI/CD for data engineering. Beyond testing code functionality, pipelines must validate that data meets quality expectations at each stage. This includes checking for expected values, relationships between fields, completeness of records, and adherence to business rules.
When data validation fails in production, data pipelines need mechanisms to prevent problematic data from affecting downstream systems. This might involve quarantining suspicious records for manual review or applying fallback transformations that maintain system stability.
How CI/CD Improves Pipeline Design
Adopting CI/CD practices naturally drives improvements in pipeline architecture. When teams need to automatically test and deploy their pipelines, they often discover that monolithic, tightly-coupled designs are difficult to test and maintain. This realization leads to more modular pipeline designs with clear separation of concerns.
Modularity becomes essential as teams break large pipelines into smaller, testable components. Each component has a specific responsibility—extracting data, transforming it according to business rules, loading it into target systems—with well-defined inputs and outputs. This modularity makes pipelines easier to test, modify, and troubleshoot.
Scalability improves as teams design pipelines to handle varying data volumes across environments. CI/CD encourages teams to test with representative data scales, revealing performance bottlenecks before they impact production. This leads to architectures that can scale resources based on workload demands.
Adaptability increases as teams implement CI/CD-friendly approaches to handle changing requirements. Rather than hardcoding transformation logic, they develop configurable pipelines that adapt to different data sources, schemas, and business rules. This configurability allows pipelines to evolve without requiring complete rewrites.
Testability becomes a core design principle. Teams structure pipelines to allow injection of test data, isolation of components for unit testing, and verification of outputs against expected results. This testability ensures that changes can be validated automatically before deployment.
"Shift Left" and "Shift Right" in CI/CD
In the context of CI/CD, two core strategies often come up: shifting left and shifting right. For data teams, both are essential but they serve different stages of the development and deployment lifecycle.
Shifting left means testing early, while development is still in progress. Instead of waiting until code is deployed or pipelines are live, teams test as much as possible during development. This includes unit testing transformation logic, validating SQL queries against database schemas before deployment, and performing early data profiling to identify potential issues. The goal is to surface issues before they reach production, when they're harder and costlier to fix.
Shifting right focuses on what happens after deployment to production. It's about building operational resilience: monitoring pipeline runs, handling failures gracefully, and adapting to real-world runtime conditions. Workflow orchestration tools like Prefect ensure that pipelines run reliably in production, with built-in scheduling, monitoring, and failure handing capabilities. These capabilities help teams identify runtime issues early and respond quickly, turning production environments into a source of operational insight.
Prefect offers parameterization capabilities, which helps teams shift right while keeping pipelines flexible. Instead of hardcoding logic for each use case, teams can define a single pipeline template that adapts dynamically based on input values. This makes it easy to run the same pipeline for different customers, time ranges, or different sources without touching the code.
In addition, Prefect's work pools manage infrastructure dynamically. A pipeline can run on lightweight resources in development and automatically scale to performance compute in production. This solves one of the key CI/CD challenges for data pipelines: managing environment-specific infrastructure requirements. Teams no longer have to manually reconfigure environments to mirror production needs; instead, the infrastructure adapts based on context, letting pipelines behave consistently across stages.
Together, these "shift left" and "shift right" practices bring CI/CD full circle for data science and data engineering. Testing earlier prevents known issues. Orchestration later ensures resilience when unknowns inevitably emerge. The result is a continuous feedback loop, one where pipelines evolve safely and teams can deploy with confidence, knowing both their code and their operations are built to handle change.
Building Your First CI/CD Pipeline
Implementing CI/CD doesn't require adopting all practices at once. Teams often start with Continuous Integration by automating builds and basic tests. This provides immediate benefits by catching integration issues early.
As the team gains confidence, they can add more comprehensive testing and automate deployments to test environments. Eventually, they might implement full Continuous Delivery or Deployment, automating the entire path to production.
The tools supporting CI/CD have evolved to make implementation more accessible. Popular CI/CD platforms like Jenkins, GitHub Actions, GitLab CI, and CircleCI provide ready-to-use frameworks for building pipelines. These tools integrate with source control systems, test frameworks, and deployment mechanisms to create cohesive workflows.
The Cultural Dimension of CI/CD
While CI/CD involves technical practices and tools, its successful implementation ultimately depends on cultural adoption. Teams must embrace frequent integration, comprehensive testing, and automation as core values.
This cultural shift often requires changes in how teams work. Developers need to write testable code and create automated tests alongside features. Teams must prioritize fixing build and test failures immediately. Deployment processes need to become routine rather than exceptional events.
The benefits of this cultural change extend beyond technical improvements. Teams experience less stress around releases, spend less time debugging integration issues, and deliver value to users more quickly. The increased confidence in code quality allows teams to innovate more boldly, knowing their safety nets will catch potential issues.
Measuring CI/CD Success
Measuring the impact of CI/CD helps teams refine their approach. Key metrics include deployment frequency (how often code reaches production), lead time (how long it takes for a change to go from code to production), change failure rate (how often deployments cause issues), and mean time to recovery (how quickly issues are resolved).
Improvement in these metrics indicates a healthier software delivery process. Teams deploying frequently with short lead times, low failure rates, and quick recovery times can respond rapidly to user needs while maintaining system stability.
For data engineering teams, additional metrics around data quality and pipeline reliability become important. These might include the percentage of data passing validation checks, the frequency of pipeline failures, and the time required to address data quality issues.
Conclusion
CI/CD represents an evolution in how software teams deliver value. By automating integration, testing, and deployment processes, teams can develop more reliable software more rapidly. For data engineering teams, adapting CI/CD practices to their unique challenges enables the reliable delivery of data pipelines that transform raw data into valuable insights.
CI/CD isn't a switch you flip, it's a discipline you grow into. Teams start with basic automation and expand their practices as they gain experience and confidence. Along the way, they develop not just technical capabilities but a culture of quality, collaboration, and continuous improvement that forms the foundation for long-term success. Most importantly, the discipline of CI/CD naturally pushes teams toward better-designed systems that are modular, scalable, and adaptable—creating a virtuous cycle of improved quality and productivity.
Further Reading
For more information check out Prefect’s documentation, or join our Slack community to get personalized support.
Related Content








